In this article
Summary
Artificial intelligence is central to the development of modern health care. Whether for treat mentor research, where its applicability is vast (research on drugs, virtual patients, etc.), AI is now an everyday technology that continues to change how we practise. Its development involves the wide-scale use of patients’ personal data. However, this leads to a paradox because the development of an AI algorithm is subject to rules concerning personal data protection, rules which were adopted when AI was more of a concept than a reality.Since then, have the current regulations* been adapted to modern forms of AI? Are AI regulatory projects condemned to becoming obsolete by definition, in light of the exponential evolution of AI algorithms?
AI and regulation: two different tempos
The General Data Protection Regulation, known as the GDPR, was adopted in 2016 and came into force in May 2018. Preparations began in 2012 with the European Commission’s proposal for a reform. The GDPR then replaced a previous Directive which was twenty years old and had come into force well before the digital revolution.
The most recent or upcoming European legislation(EU AI Act, Data Governance Act) concerning the development of health algorithms refers to the GDPR for the protection of health data used in this context.Thus, the GDPR, which is nevertheless almost 10 years old taking into account the years when it was being drafted, will remain the reference standard for years to come. This issue is also found across the Atlantic with the AmericanData Privacy and Protection Act, a US GDPR project which does not currently set out any specific implementing measures for AI algorithms.
In parallel to this long process of enacting legislation, AI itself has been developing extremely quickly.
Before the 2010s, artificial intelligence was mainly based on expert systems. In the field of health care, for example, the expert system MYCIN was created by Stanford University back in 1972, and all rules were coded by humans, based on an interference engine to diagnose blood diseases. Around 2010, the development of AI gained new momentum thanks to the combination of the volumes of data available and to the power of graphics card processors that allowed these large volumes of data to be processed. AI then saw a paradigm shift. The expert systems gave way to inductive learning systems, in which the rules were no longer coded but instead deduced by correlation and by classification of massive quantities of data. Since then the algorithms have become“self-learning”.
Over the course of its development, AI has become a ground-breaking innovation, having consequences for individuals that call for regulation by the public authorities. Inevitably, this legislative process can only be developed over a long period of time.
In fact, it is only natural for the law to intervene after innovation as it reacts to existing situations. The challenge thus lies in finding a balance between the technological and scientific progress that can be hoped for in a given field on the one hand and the containing of this progress by regulation on the other. Such containment will be justified by the need to avoid abuses linked to technological progress[1].
The law must therefore be specific enough to contain the existing practice but also broad enough not to stall progress.
From theory to practice, the difficulties of applying regulation to artificial intelligence algorithms
The GDPR sets out the principles to be followed by every company that processes personal data. These principles have a strict interpretation and stronger safeguards when it comes to health data, which is known as sensitive data. When data processing concerns AI algorithms in health care, there are enormous challenges.
Firstly, there is not one but many AI solutions. Depending on the AI model developed, the practical consequences for complying with the regulation are very different. Therefore, an AI solution embedded in a medical device does not have the same restrictions as a so-called“SaaS” or Software as a Service solution in the cloud. The challenges of data security are different because differences in development and use trigger solution-specific vulnerabilities.
Furthermore, the methods for developing anAI algorithm also differ themselves. The federated learning method where the algorithm is used directly at the data provider is distinct from a LargeLanguage Model known as LLM (ChatGPT, Bard, etc.) which is trained on large datasources that are diverse and for which compliance cannot be verified. A federated learning model presents additional safeguards, as the source data do not pass between the data provider (i.e. hospital) and the final user (i.e.manufacturer). However, these techniques are not without risks. In fact, the company developing the algorithm is dependent on the security of the data provider and this leads to the risk of “data poisoning” (modifying a model at source by working on falsified data) [2].
In addition, the relevance of the scientific results is also called into question, particularly when using LLMs.The recent initiative of an American doctor who sent ChatGPT the clinical cases of eight patients who had been admitted to the emergency department speaks for itself. AI was unable to give the correct diagnosis in two cases. Two patients whose lives would have been put at risk [3],due to the incorrect diagnosis by the algorithm, had the doctor followed the AI’s decision.
It is also necessary to ensure that these multiple AI models share the same degree of compliance with the general principles set out in the GDPR.
For example, the principle of data minimisation, which involves only processing the data necessary for developing an algorithm. In practice, it is difficult to pre-define the necessary data fora self-learning model where the mechanisms do the “reasoning”, and therefore the learning cannot be explained by its actual creators (the famous “black box effect” of deep learning models).
We can add to this the further challenges linked to “data curation”, i.e. cleansing data prior to model development. We can use the example of an AI model helping with diagnosis in radiology, which represents three quarters of submissions of solutions involving AI to the FDA[4]. In this case, should we, as required by the legislation, delete all the metadata associated with each of the files? If yes, the human burden and financial cost become substantial regardless of the size of the company processing the data.
The issue of patients’ informed consent is also another sensitive subject in the context of their data being used by AI.Frequently, consent is used or even required as the legal basis for processing health data. So how do you ensure you have the informed consent of a patient whose pseudonymised data (without any direct identifying information) is being reused by a company unaware of their identity? Furthermore, is such consent really desirable from the point of view of medical research, insofar as it concerns the use and reuse of the data of each individual and not each database?
Here is an example to illustrate the point:a company developing an AI algorithm wants to reuse the data of 100 patients to train its algorithm. If consent is chosen as the legal basis for data processing, this company is dependent on the individual consent of each patient to be able to use their data. Even if it were possible to directly contact these patients, through a digital solution involving their telephone, how can it be guaranteed that these 100 patients will respond to the request for consent? The company in question may therefore only be able to reuse the data of some of the patients if only a limited number of them respond. In any case, strictly applying the law would seem to limit or even jeopardise the AI development.
Faced with this, it is worth identifying the existing empirical solutions and more structured future solutions.
Are legal and practical innovations enough?
In light of the presented challenges, there are three different solutions:
Firstly, the authorities that oversee such issues have a part to play. They are in contact with the companies developingAI. They can publish codes of conduct and guidelines to accompany the application of the GDPR to AI, while effectively adapting to technological advances. As such, the French, Spanish and UK data protection authorities have issued guidelines [5]on AI development. On the other side of the Atlantic, the FDA authorised 115 medical devices embedding AI in 2021. 96% of these submissions are also made under 510(k), which exempts applicants from a “clinical trial” or a similar approach if the device is substantially equivalent to an officially marketed device which has already been assigned a class. Nevertheless, the FDA has created the Digital Health Center of Excellence to modernise the authority’s regulatory approach to AI. In particular, it is expected that in the future an AI developer will have to provide a “change control plan”, in order to document how the algorithms evolve after they have been marketed and to guarantee their performance.Finally, at international level, the G7 of data protection authorities is working on a code of conduct for generative AI (i.e. ChatGPT), in particular to regulate the use of source data that are compatible with current regulations[6].
Secondly, companies developing AI must now integrate the protection of individuals’ data into their development. In this respect, recent awareness seems genuine as evidenced by the demand for a legal framework from the major players in AI [7]. This need for a legal framework goes beyond data protection, which nevertheless remains an essential component for trusted AI. From now on, companies developing AI must document, in particular through risk analysis, any uncertain or unknown elements jeopardising their compliance.
In light of these new obligations, innovative and pragmatic solutions may be developed to limit the risks. TheCompliantGPT initiative has therefore been proposed to act as an OpenAI interface where the health data concerned is replaced with a token. This solution could enable compliance with the US HIPAA standard [8]. In any case, it seems clear that the authorities, faced with these uncertainties, expect the companies who are developing AI to come up with proposals to limit the risks related to personal data protection.
Lastly, the law will intervene retrospectively to legitimize or invalidate the uses established by the players in the sector. In this respect, the European Union is a pioneer in the field with itsEU AI Act. The latter refers to the GDPR for data protection but also sets out measures directly concerning its application. In particular, the EU AI Act formalises the obligation to prevent algorithmic biases for high-risk AI, including AI in health care[9].
This issue of algorithm bias is central to how much trust we place in AI solutions as the consequences such bias can have on patients may be extreme.
In conclusion, the race between innovation and the law may seem to be lost before it has even started. Nevertheless, the law is not the be all and end all of compliance and development, and it is up to intermediary bodies to define practical rules of use for personal data in this context. Recent advances show that there is a consensus on the need for regulation. We believe in a flexible approach to regulation based on commonsense. This is the philosophy we apply on a daily basis in our dealings with clients and the data protection authorities.
*This article only deals with the regulations on personal data protection and therefore does not mention the challenges of medical liability, which are also very relevant, or the practical issues of data interoperability.
[1] Nature– Bias in AI-based models for medical applications: challenges and mitigationstrategies
[2] IAPP– Federated learning supporting data minimization in AI
[3]I’man ER doctor: Here’s what I found when I asked ChatGPT to diagnose my patients
[4] 5takeaways from the FDA’s list of AI-enabled medical devices
[5] Frenchauthority guidelines, Spanishauthority guidelines, UKauthority guidelines
[6] G7data protection authorities point to key concerns on generative AI
[7]MicrosoftCTO Kevin Scott thinks AI should be regulated
[8] UsingChatGPT and Generative AI in a HIPAA-Compliant Way
Sign up for our newsletter
We like to keep our readers up to date on complex regulatory issues, the latest industry trends and updated guidelines to help you to solve a problem or make an informed decision.
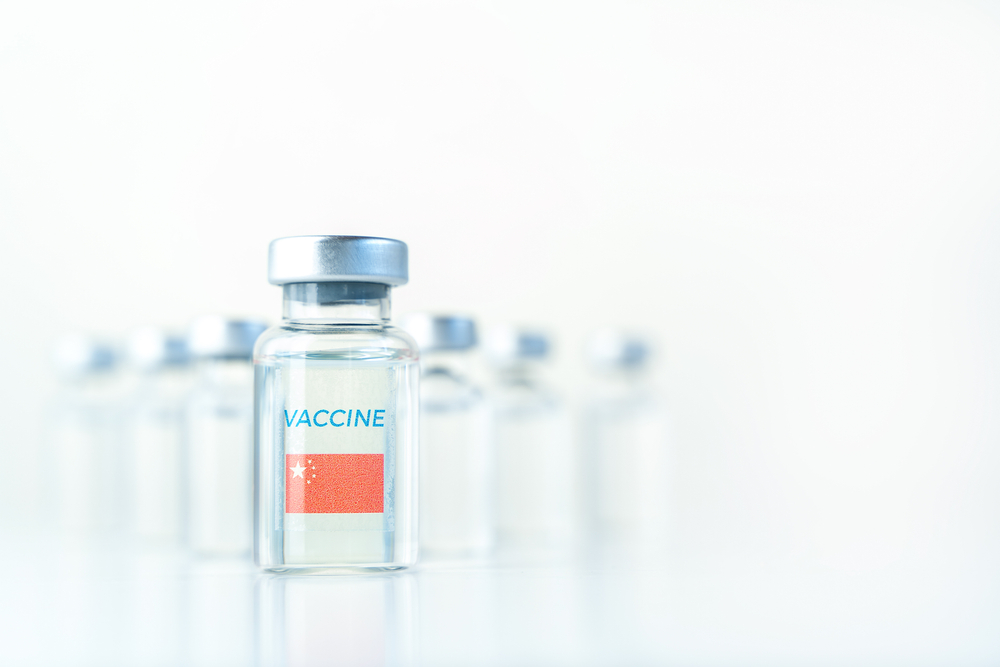
Navigating Privacy Requirements for Clinical Trials Across Jurisdictions: Focus on China
China’s data protection regulations play a crucial role in clinical trials, requiring sponsors and researchers to comply with multiple laws, including the PIPL, GCP-2020, and cross-border data transfer rules. Unlike other jurisdictions, China imposes strict consent requirements, risk assessments, and regulatory filings, making compliance a key factor when selecting trial locations and managing participant data.
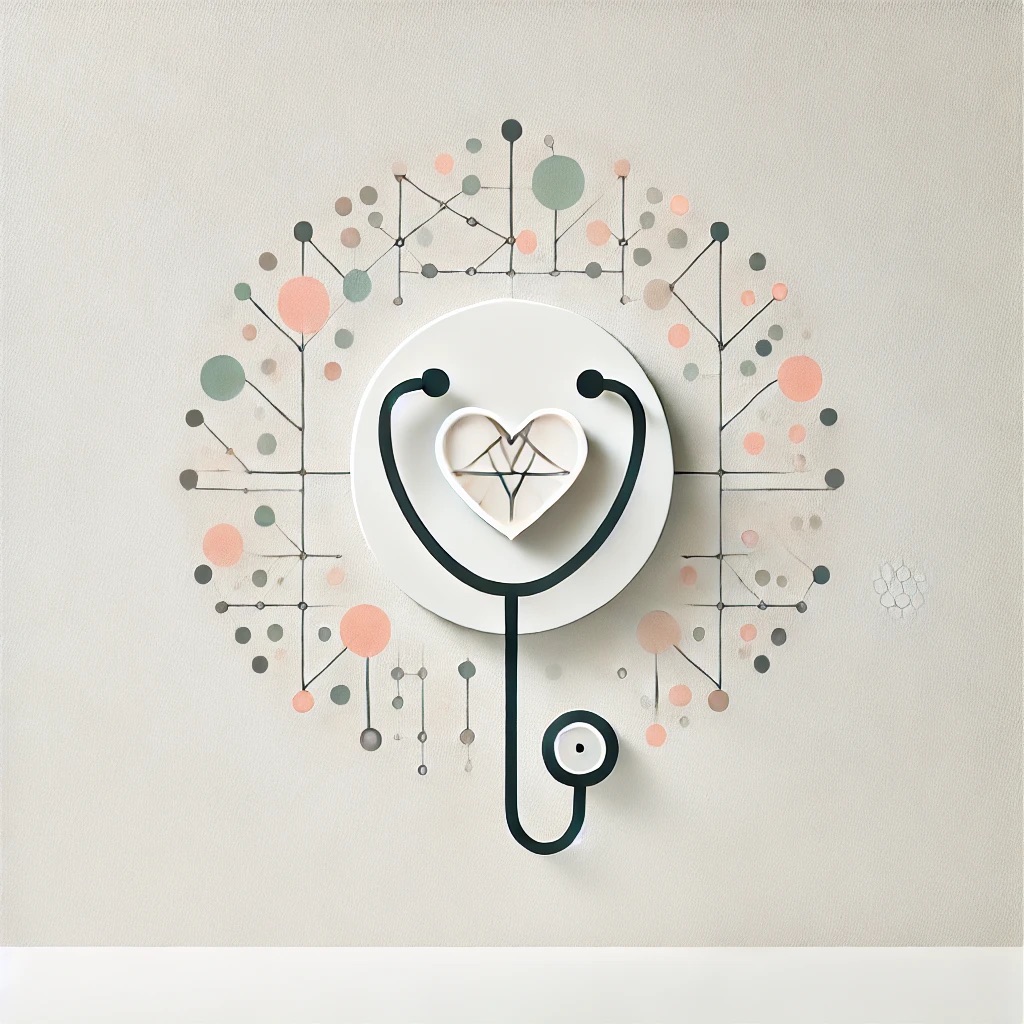
Addressing the Data Protection and Ethical Challenges posed by AI in Health – Part I
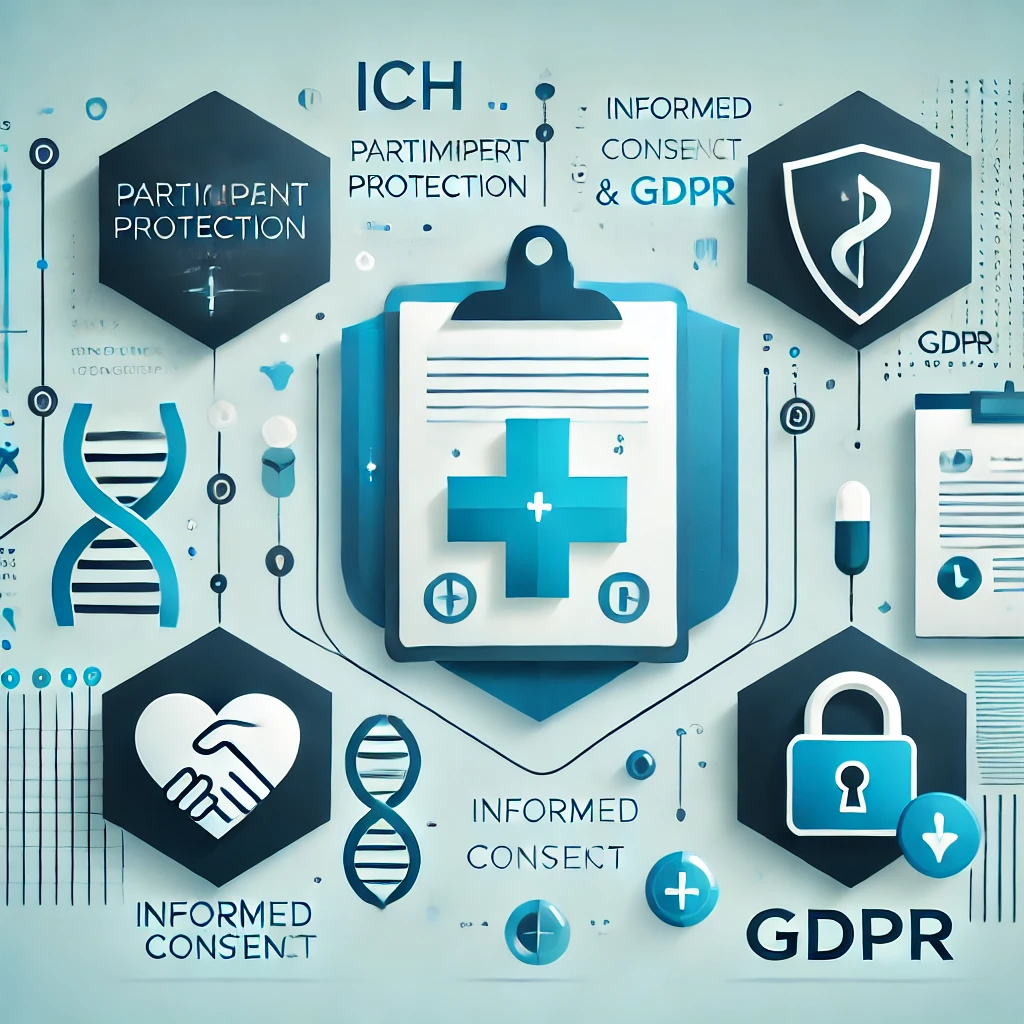
Analyzing the Similarities and Differences Between ICH-GCP and GDPR in Clinical Trials
ICH-GCP and GDPR are vital for clinical trials, setting standards for participant protection and data integrity, with distinct focuses and enforcement approaches.