In this article
Summary
Artificial Intelligence is revolutionizing Life Sciences with unparalleled power. It offers great opportunities to improve health care.However, the volume of data needed to train key models of AI research and application raises ethical and regulatory questions. In order to protect patients’ health data, it is crucial to guarantee a virtuous and transparent exploitation of Artificial Intelligence models. In this constantly and rapidly evolving context, what measures should be put in place to comply with the regulations in force and secure companies and healthcare professionals in the use of data?
AI at the service of prodigious advances
The promise of Artificial Intelligence(AI) in this industry is prodigious. AI plays an increasingly important role in this because of its ability to process large amounts of data from multiple datasets and make them interoperable.
In fact, AI can have compenetration at every stage of the pharmaceutical industry’s value chain, from the molecule to the market. Used to improve molecular research and clinical trials, AI also optimizes the time to market of therapies, the supply and manufacturing chain, and healthcare professionals’ engagement with patients.
While Machine Learning algorithms make them able to make decisions or make predictions on new data, Large Language Models (LLM)go further and open the possibility of prescriptive with reasoned solutions.These engines, capable of analyzing complex and heterogeneous data, providing a first response to the difficulties of interoperability of health data,are now able to infer. By developing deductive reasoning, they can identify patterns and correlations that might not be detected by traditional methods of analysis.
Foundation Models help overcome some of the challenges facing current health systems, such as healthcare inequalities and shortages of healthcare professionals.
Indeed, the intelligent technologies of Foundation Models have the potential to understand a context. They offer the opportunity for healthcare practitioners to make more informed decisions, based on real-time data and more accurate predictive models. They enable more efficient use of data and significantly reduce the cost of training specific models.
The applications are multiple and impactful:
· Molecular research
By applying data science and machine learning to big data sets, AI is transforming the search and discovery of new molecules. For example, AI can help analyze data from genomic sequencing, microcopy, or other research tools. In particular, it can produce a prediction of protein structure using DeepBlue’s databases of protein structures and millions of three-dimensional configurations. From the amino acid sequence, using artificial neural networks (Machine Learning models that mimic the functioning of the human brain), the researchers developed and manufactured a protein that does not exist in nature and that aggregates several antibodies ina sphere to boost their action to make COVID-19 vaccines more effective.
· Drug development
AI helps accelerate the drug development process by identifying therapeutic targets, predicting the pharmacological properties of candidate compounds, optimizing dosing regimens ,and predicting potential side effects. The first AI-powered drug entered clinical trials in record time. Only 12 weeks were enough for the British start-up Exscientia, accompanied by the Japanese company SumitomoDainippon Pharma, to find a molecule that cures obsessive behavioral disorders.
· Clinical trials
Continuous streams of clinical trial data can be cleaned, aggregated, coded, stored, and managed. The automation of study protocol generation and the acceleration of manual tasks are made possible by natural language processing. IA can significantly reduce cycle times and costs of clinical trials, while improving clinical development outcomes. For example, failed protocols can be analyzed to assess the risk of failure and identify which parts of a new protocol are problematic. Improving these processes could speed up the time to market the new drugs and reduce research costs and thus their selling price. It is estimated that about 85% of clinical trials aimed at developing new treatments fail before being approved by the European or US drug agencies.
· Personalized medicine
AI can help healthcare professionals quickly and accurately diagnose diseases by analyzing patient data, including medical images and lab test results. It can lead to significant improvements in personalized medicine. Providing relevant details to support informed and timely decisions about patient treatment can in fact lead clinicians to better understand the mechanisms of treatment of certain diseases. It is in this sense that Europe is deploying thePerMedCoE programme (HPC/Exascale Center of Excellence in Personalised Medicine). For the personalization of cancer treatments, Institut Curie (France) teams use AI supercomputers to model all the interactions between the different actors of an organ, at the genomic, proteomic, and metabolic levels. The hope to quickly decipher the complexity of the data, understand the evolution of a tumor, and offer a specific individualized treatment.
· Patient-physician relationship
In addition, Foundation Models can be used to automate certain tasks related to patient management, such as scheduling appointments and writing reports.
AI can help doctors free up valuable time to spend with patients by simplifying many daily tasks. AI also facilitates communication between healthcare professionals and patients with, for example, medical chatbots or mobile apps to provide advice and health information to patients. The Goodmed application centralizes reliable data on each drug and delivers personalized information based on health information recorded by the user. A simple scan of the drug by the patient makes it possible to adda level of safety to the taking of the treatment, to know the adverse effects or to avoid misuse.
AI can also analyze patient comments and complaints, medical inquires, and social media data to incorporate patient voice into product iterations.
· Supply and manufacturing chain
By applying AI to biopharmaceutical manufacturing facilities and processes, stakeholders can predict and identify quality control issues and proactively suggest corrective actions to minimize disruption and improve product distribution.
With the Area platform, global pharmaceutical companies, such as the Merck Group, can benefit from real-time representation of their entire operational level. AI is able to build analytical models and recommendations based on use cases, such as quality and delivery time management even within the framework of complex infrastructure. This breakthrough allows forecasters to focus on the most important decision-making.
· Epidemiological surveillance
Real-time monitoring of epidemics and pandemics, analyzing data from various sources such as social media, media reports and geolocation data, is made possible. Bluedot usesAI and data analytics to monitor outbreaks and infectious diseases. TheCanadian company detected and reported the COVID-19 outbreak in China as early as December 2019 as well as emerging infectious disease outbreaks around the world. Alerting institutions and enabling them to take preventive measures is not the only ambition of Bluedot which has also provided risk analyses related to the pandemic to help travelers, businesses and organizations make informed decisions.
An already obsolete regulation?
If technology promises progress, it is also a source of questions to guarantee respect for fundamental rights and human security.
The regulation aims to regulate intelligent technical processes to guarantee their efficiency, transparency, fairness, non-discrimination and the protection of personal data and privacy.
The European texts place a series of prior obligations upstream of AI developments: precise definition of the scope of an AI, registration, documentation, traceability, governance, approval audit, European conformity, and data protection labelling, etc.
In practice, implementation is complex. AI is constantly evolving and can exceed certain established limits. For example, traceability, this idea that we will try to understand how an artificial intelligence comes to suggest an action, or even to make an effective decision. Machine learning algorithms are constantly learning and evolving, making it difficult to regulate their behavior.
In Life Sciences, AI raises important issues in terms of regulation and data privacy. Health data is considered sensitive information and is therefore regulated by the Personal Data Protection Act, or GDPR, in Europe. The right to be forgotten is an important principle of personal data regulation, which allows individuals to request the deletion of their personal data. However, with the increasing involvement ofAI, is it really possible to completely delete data stored in machine learning models? Similarly, informing individuals individually of the use of their data to train an AI model seems almost impossible to implement in the event of data reuse.
AI algorithms using LLMs can be extremely complex and difficult to understand. It can be difficult to determine how these algorithms make decisions and how they arrive at their results.
Therefore, should validation and reliability standards be put in place to control the black box effect and ensure that AI results are accurate, reliable, and reproducible?
The current regulation does not fully cover the use of AI in healthcare, which requires an update of existing regulations to address the issues raised by AI and LLMs.
Is it possible to deal with all situations?
The use of AI raises complex regulatory issues that must bead dressed to ensure compliance with applicable rules and regulations.
Intellectual property protection issues are also raised in the context of new drugs or health products. Are the algorithms and AI models used considered patentable inventions?
In the face of AI, companies need to address these questions and work with regulatory and legal experts to ensure compliance.
Ensuring ethical exploitation
The challenges of using these models responsibly and ethically are significant. They include the quality of the data used to train the models, the need to ensure that the models are not biased towards certain populations or diseases, and the need to ensure the confidentiality and security of the health data used to train the models.
The ethical component of AI addresses issues of accountability and transparency.It must be ensured that decisions made by AI systems are not discriminatory or biased and that the rights of individuals are respected. This expertise includes understanding human and algorithmic bias, designing fair and transparent AI systems, and establishing redress mechanisms for individualswhose rights have been violated.
Organizations need to be transparent about how AI works in the processing of health data and provide clear and easy access to information.Patients must be informed about how their health data is used and have the right to know how medical decisions are made about them. Institutions and regulators must ensure that companies adhere to the highest ethical standards.
One can only advocate for an open dialogue between policymakers, healthcare practitioners, AI researchers and patients to ensure that AI used ethically, effectively, and safely in healthcare.
Multidisciplinary expertise
In short, the use of AI and LLMs in Life Sciences represents a unique opportunity to improve healthcare. While it is important to continue to explore the benefits of AI in Life Sciences, it is essential to ensure that the privacy and security of health data is protected. To do this, the current regulations need to be reviewed and adjusted to these innovations.
Ensuring compliance with applicable regulations, transparency and accountability are the key elements to ensure the ethical use of these technologies that require multidisciplinary expertise, ranging from personal data regulation to data governance.
The keys to good practices can be summarized as follows:
· In-depth knowledge of personal data protection regulations, such as the GDPR (General DataProtection Regulation) or the Data Governance Act in Europe is necessary to understand the legal obligations and compliance requirements for companies and organizations that use personal data.
· Data governance expertise is required to ensure that data is collected, stored, processed, and shared ethically and responsibly. This includes establishing clear and transparent privacy policies, identifying, and managing data privacy and security risks, and defining clear processes for gaining buy-in from individuals for the use of their data.
· Technical expertise is also required to implement technical solutions to protect personal data, such as anonymization ,encryption and pseudonymization. Technical experts need to understand legal data protection requirements and industry best practices to ensure that technical solutions are effective and comply with data protection standards.
· Expertise in AI ethics is essential to ensure that decisions made by AI systems are not discriminatory or biased and that the rights of individuals are respected. This expertise includes understanding human and algorithmic bias, designing fair an transparent AI systems, and establishing redress mechanisms for individuals whose rights have been violated.
iliomad supports your AI projects
iliomad’s expertise aims to help biotechnology, pharmaceutical and medical technology manufacturers comply with healthcare data protection regulations and protect sensitive patient and user data, to minimize the risk of data breaches and preserve their reputation and legal compliance.
Sign up for our newsletter
We like to keep our readers up to date on complex regulatory issues, the latest industry trends and updated guidelines to help you to solve a problem or make an informed decision.
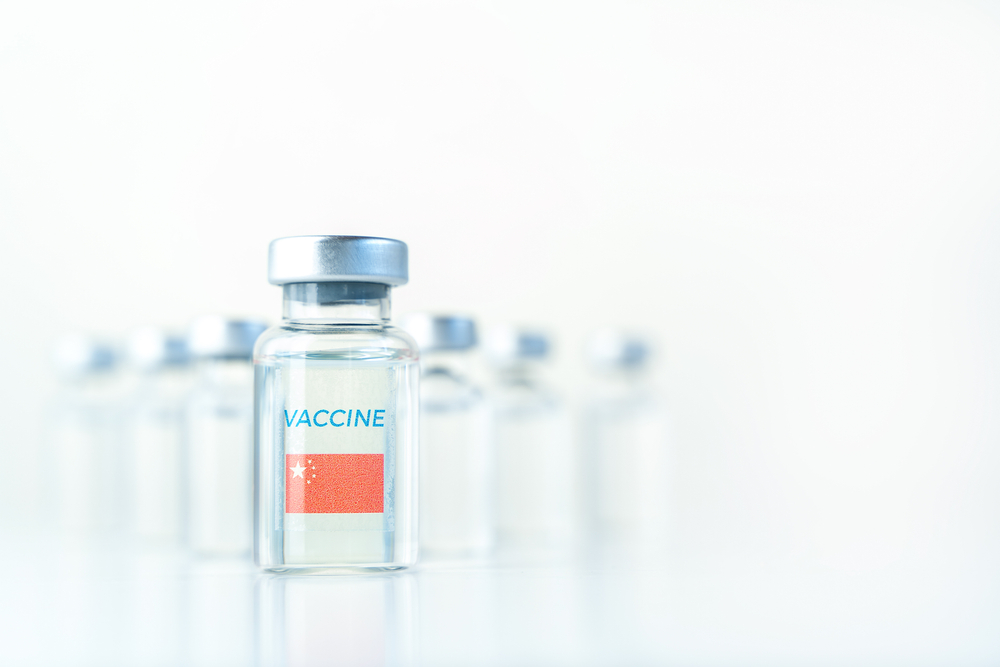
Navigating Privacy Requirements for Clinical Trials Across Jurisdictions: Focus on China
China’s data protection regulations play a crucial role in clinical trials, requiring sponsors and researchers to comply with multiple laws, including the PIPL, GCP-2020, and cross-border data transfer rules. Unlike other jurisdictions, China imposes strict consent requirements, risk assessments, and regulatory filings, making compliance a key factor when selecting trial locations and managing participant data.
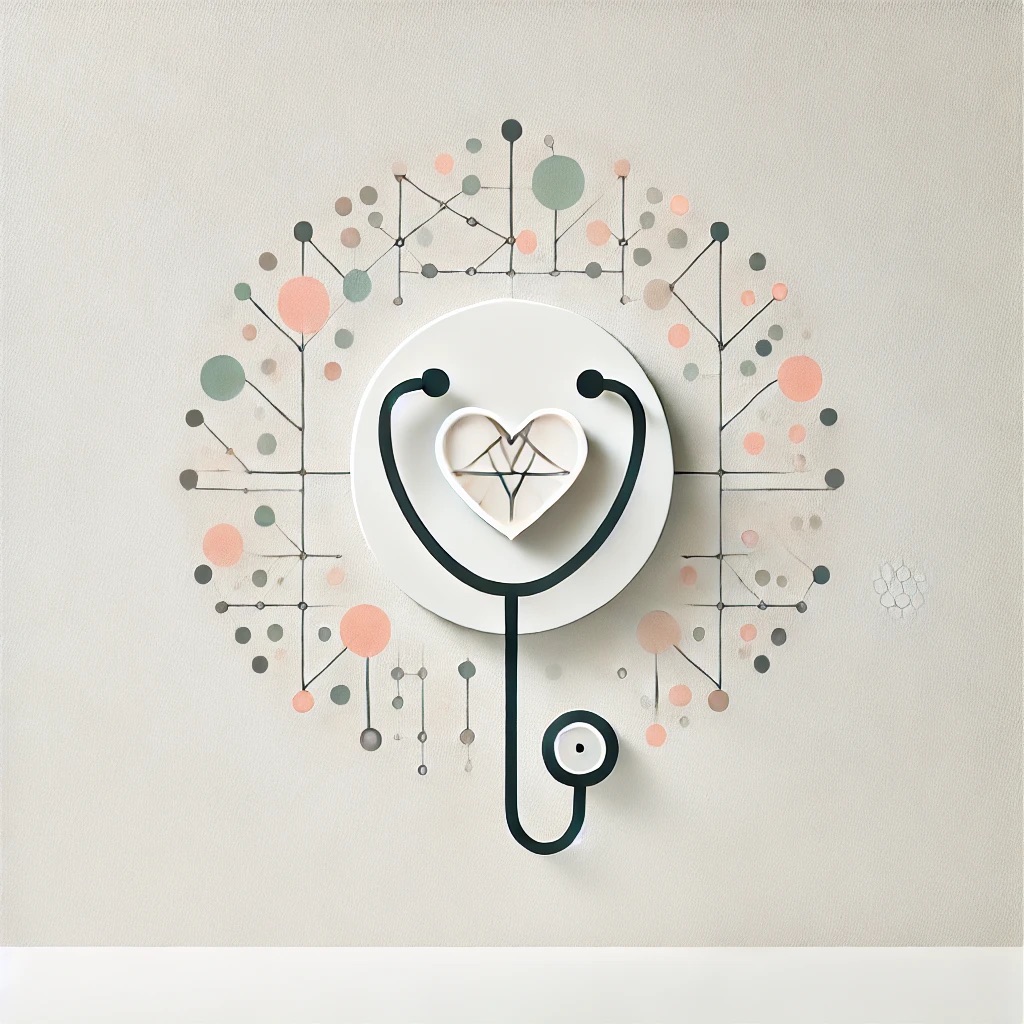
Addressing the Data Protection and Ethical Challenges posed by AI in Health – Part I
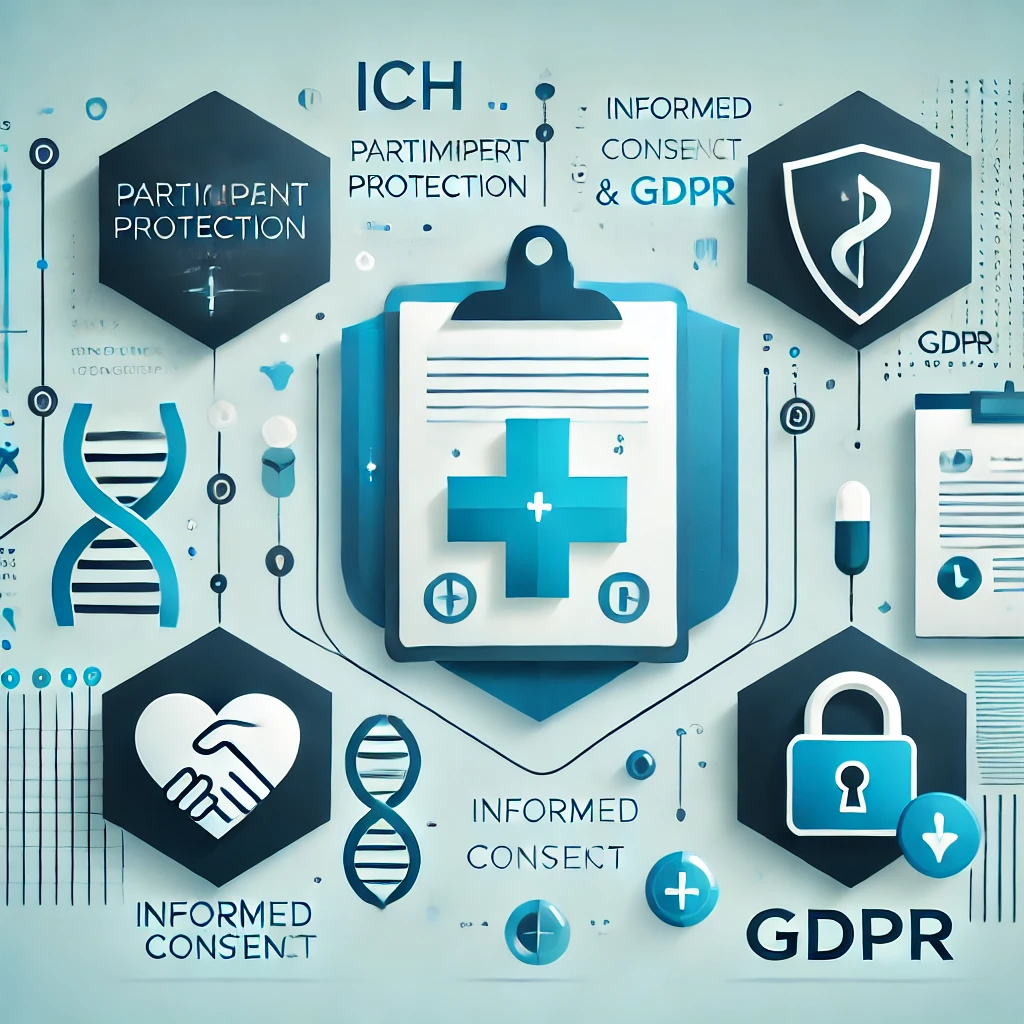
Analyzing the Similarities and Differences Between ICH-GCP and GDPR in Clinical Trials
ICH-GCP and GDPR are vital for clinical trials, setting standards for participant protection and data integrity, with distinct focuses and enforcement approaches.