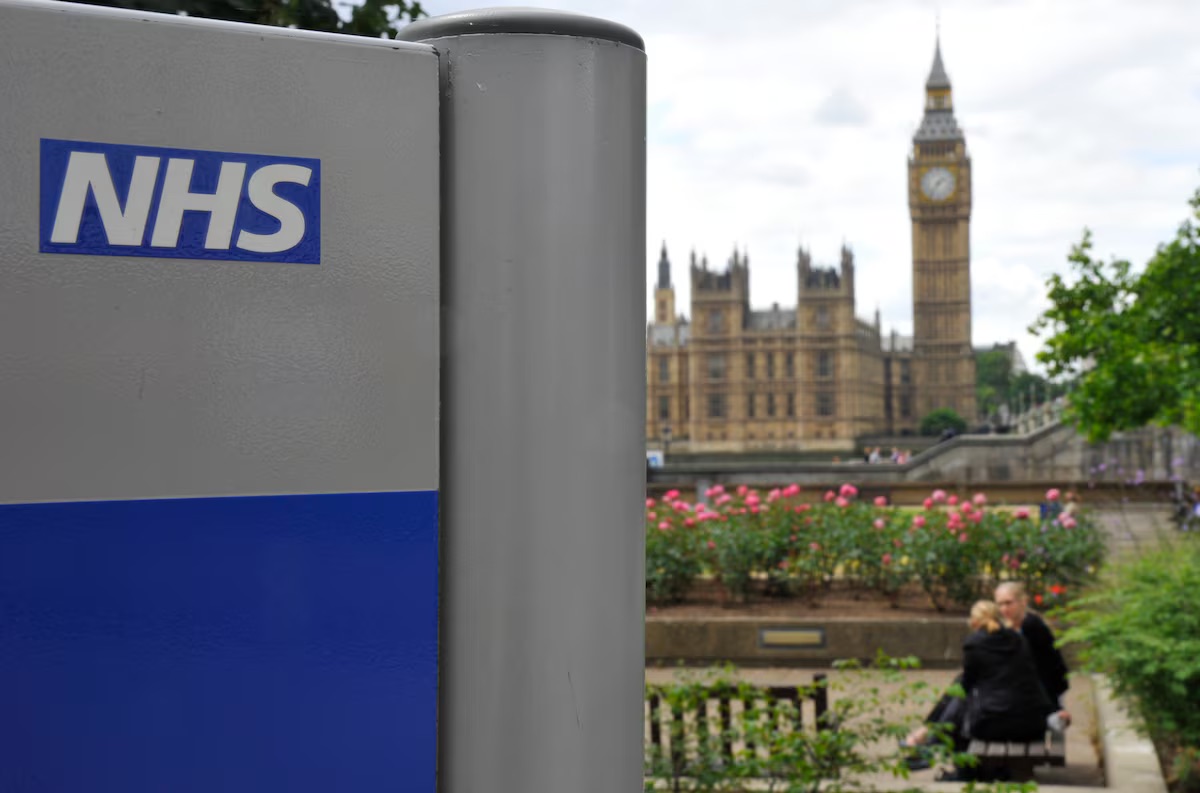
June 25, 2024
No items found.
UK's NHS says hackers have published data stolen in ransomware attack
The UK's National Health Service (NHS) has confirmed that data stolen in a ransomware attack on Synnovis, a medical diagnostics service, has been published online, and the extent of the breach and its impact on patients is under investigation.

April 29, 2024
Regulation
FTC Completes Updates to Health Breach Notification Rule for Health Apps
The Federal Trade Commission announced it has finalized changes to the Health Breach Notification Rule (HBNR) that will strengthen and modernize the rule by clarifying its applicability to health apps and other similar technologies and expanding the information that covered entities must provide to consumers when notifying them of a breach of their health data.